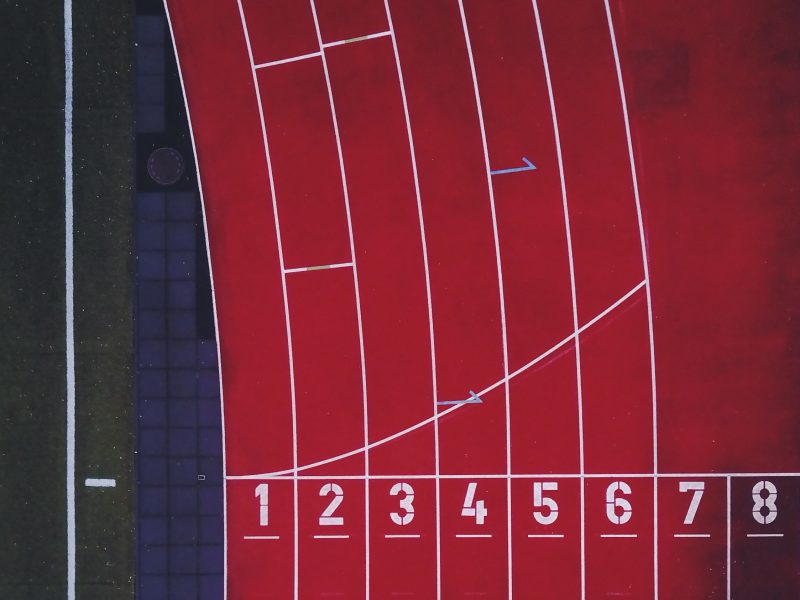
Getting started with your Optimization Strategy
This post is an edited transcript of our recent conversation with Nick So, Director Optimization at WiderFunnel on our Masters of Growth Podcast.
Use a framework for generating hypotheses
The first thing that you’ll need if you want to optimize is a framework for coming up with ideas on elements to test and modify. In other words, to come up with decent hypotheses, you need to have the right mix of creativity and data comprehension strategies.
It’s not a matter of just churning out ideas like spaghetti, throwing them at a wall and hoping they stick. You’ll need a sense for which insights are useful, both in the ideation phase and in the analytical phase.
It is very helpful if you have some sort of framework or process in place, so when you gather data whether user research, data analytics, or results from past experiments, you can turn them into actionable insights and generate new strategy points.
One example of this kind of framework is the WiderFunnel Infinity Optimisation Process:
The folks at WiderFunnel divide the optimization approach into two parts: the creative phase and the validation phase. In the creative phase, the idea is to search for insights from analytics, look at user research, persuasion, and marketing principles, and consider consumer behavior, taking into account the business contacts and business goals. All this information is collected and sorted, then plugged into the next phase for validation.
The validation phase is all about experimentation: everything should be confirmed or dismissed by data. All these insights from the creative side are examined and validated using actual tests and hypotheses.
The third part of this framework is the LIFT model, which may be familiar to CRO enthusiasts. It basically weighs the positive characteristics of a page against the negative characteristics.
What the LIFT Model aims to do is to translate all these different data points, from marketing psychology or data analytics to user research, and to filter all those different elements into a common language that everyone across the organization can comprehend. When a designer says, “I don’t like how this looks,” the statement is not yet actionable, even if it might be justified. What does it tell us? But the LIFT Model allows us to understand the sentiment better: perhaps the element was unclear to the user, distracting from the value proposition. So the model takes away the subjectivity marketing and forces people to be accountable for their ideas.
Don’t try to test too many things at once
Once you’ve developed a few hypotheses for testing, it is not uncommon for people to get excited about the experimentation and start running several different tests at the same time. If this is you — think about the quality of your hypotheses. If you’re trying to test too many variables at once, you could be increasing the risk of false positives. Keep an eye on statistical significance and sample sizes: that way you can avoid making mistakes by being overzealous.
Start your Optimization Program today!
Try iridion PREMIUM for 30 days free. No credit card required!
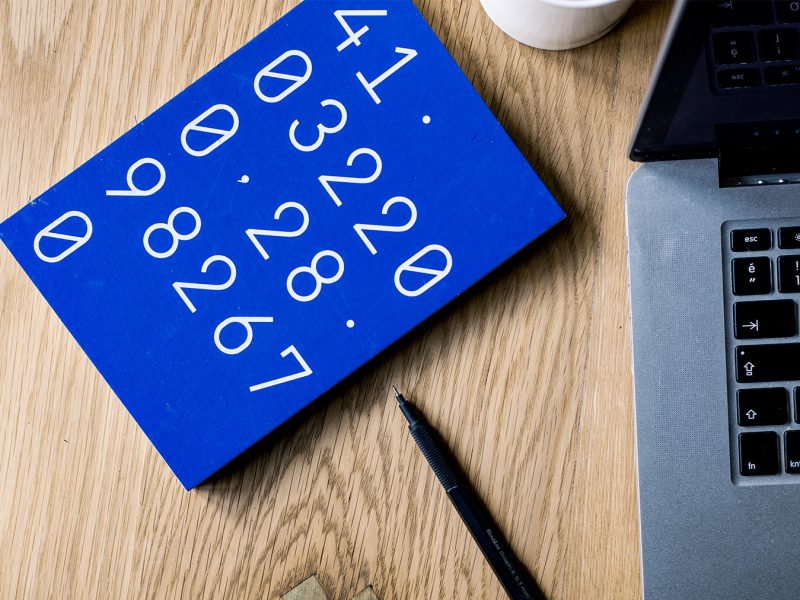
More Uplift, More Insight – 4 unseen factors in successful optimization
Every optimizer knows that a test can end with outstanding uplift one day, and the next day you’re left wondering why the last test failed. Conversion-optimization is more drudgery than the superhero images would have us believe.
Sooner or later, there are important questions that an optimizer needs to answer:
- “How can I separate bad ideas from good, early on?
- “How can I increase my rate of success?
- “How can I improve results and uplift?
Consider an ocean voyage… but on a stormy sea. It takes a stable craft, reliable navigational equipment, a hard-working crew and a captain who knows what he’s doing.
We have been at the business of sailing the “Sea of Conversion” for many years. What we know for sure is that every detail is important and every aid is relevant. To cross that sea with certainty, we’ve put together the most essential components for a successful voyage.
1. Build the boat – identify weak points and generate measures for optimization
The Alpha and Omega of any successful experiment comprises the ideas and the hypotheses at its foundation. Every successful experiment draws on a good idea or a good hypothesis at its heart. To tackle the right issue, it’s important to ask the right question.
Qualitative analysis leads to new ideas
To generate measures for optimization, important factors in addition to knowledge on the metrics for the site are creativity, empathy and a sound understanding of user behavior. Models can help guarantee continual high performance, making sure that input goes beyond mere subjective opinion and that user purchasing behavior is always being questioned.
The “seven levels of conversion” framework
Why should the seven levels framework be used?
The framework allows a simpler pick-up on the user’s decision-making process, thereby identifying weak points. You can see through the user’s eyes and comprehend a website visit from that perspective.
How do I benefit?
The weak points that are identified by using the framework offer new sources for optimization measures. If the framework is used regularly it helps to derive the right ideas or hypotheses for the particular website and target group.
We have digitalized the seven levels framework for the first time and we are making it accessible through Iridion. It has never been easier to use the framework.
2. Sail in the right direction – prioritize optimization measures the right way
Regardless of whether the issue concerns a sophisticated hypothesis or a quickly-formulated optimization idea, sooner or later the question will arise: How do you objectively evaluate and determine what’s worth testing?
Usually, assumptions that appear logical form the foundation for deciding what needs to be tested:
- How much traffic does the particular site see?
- Which sites have the highest drop out rate?
- What’s easy to implement?
- Or will the HIPPO (highest paid person’s opinion) simply once more determine how to go forward?
In reality, decisions are often made based on these or similar criteria. To reach well-founded decisions, however, it is important to use an evaluation system that is as objective as possible and one that incorporates all influencing factors that are relevant to the optimization measures.
Systems that can help in prioritizing are, for example:
- The PIE Framework by WiderFunnel
- CHPL-Scoring by konversionsKRAFT
What good will it do me?
Resources are often limited, and it is therefore particularly important to do testing only where a good cost-benefit relationship exists. The scoring provides a precise tool to isolate measures that are really worth the expense of an A/B test.
Tip:
With a system like that, personal HIPPO preferences can also be included and possibly weighted more highly. The chances of making all stakeholders happy and reaching a consensus increase enormously because of this system.
We have integrated CHPL scoring, which has been proven in practice, into Iridion and made it accessible to everyone.
3. Keep the logbook current – control your experiments
It isn’t easy, getting an overview of all of the information relevant to an experiment. But knowing what happened in all of your past and present experiments at any given time is essential in order to make meaningful decisions or to pass knowledge gained along to the right people.
The following questions should be answered in order to continually cultivate information that relates to the CRO process:
- What’s being tested?
- What is the current state of the current tests?
- Which tests were successful and which were not?
- Which hypotheses / ideas or weak points were tested?
- How do I quickly set up a results report?
Iridion helps answer these and other questions at any time.
Tools that can help organize tests (but are not directly associated with Iridion)
- Excel to prepare test results
- Powerpoint/Keynote for results reports
- Dropbox or Fileserver for Wireframes, concepts and other supplements.
- The particular testing tool for all test-related data and visualizations
- A PM Tool that coordinates and allocates the particular test status
- Google Docs for collaborative work
These solutions are not centralized and are very hard to automate, so Iridion puts everything into one solution.
Iridion makes it possible to obtain an overview at any time, and to grasp all important information prepared in one view.
4. Maintain knowledge and make it reusable – maintain the logbook
Information that results from testing is often developed with difficulty only. It is important that knowledge gained be put to use and retained after implementing the change. Every test can lead to an improvement in the foundation for follow-up decisions.
A typical scenario
“Two years ago Mr. Schulz, the responsible person at that time, had already tested a similar scenario. Unfortunately, Mr. Schultz is no longer working with us and knowledge acquired at that time is no longer available. What now?
A “CRO” Wiki
Quality and number of A/B tests are not the only factors crucial to guaranteeing continued forward movement. The application of knowledge already obtained is a relevant influencing variable to achieve a greater probability of success and an advantage over the competition.
Why do I need this?
Optimization always includes a learning curve. Optimization measures can therefore go through a number of cycles. If care is taken to avoid loss of available knowledge and, instead, that knowledge is used meaningfully, then hypotheses and test concepts can be better tuned to a particular target group.
The following tools help make knowledge permanently accessible:
- Internal Wiki for knowledge storage for test concepts (time consuming)
- Excel as storage for test results (not fit for teams)
- A PM tool can maintain and characterize knowledge in the ticket system (often ill-suited for test results)
- PDF’s with test reports on the server (not easily searchable)
Whether there is a significant uplift or not, every test still provides knowledge that should be recorded. Iridion makes the development of a CRO-Wiki possible.
Upshot – holding to a course isn’t easy
Every phase of the trip makes use of various methods and tools. Which ones– and how many of those are suitable– are often difficult to determine. In the end, the efficiency of the measures should increase and the outcome to the conversion optimization should be influenced positively.
We found it inconvenient, having to refer back to a hodgepodge of various tools during our daily work in order to master our optimization procedure.
We developed a tool that displays the complete flow of work centrally, in one solution– to simplify the CRO every day and to design it as efficiently as possible – Iridion.
Learn more about the tool here – Iridion – Welcome to our world.
Start structuring your Experiments today!
Try iridion for free as long as you want by signing up now!